Co-Authors: Kate Lewis, Libby Koolik, Julia Luongo, and Drew Hill
Low cost sensor-based monitors can provide insight into fluctuations in air quality at a local scale. The reliability and utility of these insights, however, can vary substantially between sensor makes, models, and types.
The Shair team is exploring the ability of low-cost PM2.5 sensors to reproduce measurements from regulatory grade equipment. Regulatory grade equipment describes devices that are recognized by the Environmental Protection Agency (EPA) as the national standard for consistent, reliable air quality data — such as Beta-Attenuation Monitors (BAM). So why doesn’t everyone use a regulatory monitor to measure local air quality?
These devices and their maintenance are very expensive — approximately $15,000 to $60,000 per monitor, upwards of $200,000 for initial site development for EPA compliance, and a considerable amount of employee time in operation and compliance-related expenses according to a recent presentation by a member of the Bay Area Air Quality Management District. It is not economically feasible to implement an EPA-compliant BAM on every street corner. Low-cost sensors that perform well against regulatory grade equipment open the door for everyone, including those in disadvantaged communities, to access real-time, local air quality information.
The Shair team is currently working with Groundwork Richmond to install 50 such low-cost sensor-based monitors across the City of Richmond as part of a Community Air Grant funded by the California Air Resources Board (CARB). Before we outfit this monitoring network and begin to make air quality information available to the public, we want to know exactly how the low-cost monitors perform, especially in relation to the more-expensive equipment traditionally relied upon for regulatory and health science purposes.
To do so, we partnered with the Clarity Team, which that produces the low-cost sensors deployed by Groundwork Richmond across the City of Richmond, and CARB’s Advanced Monitoring Techniques Section to test Clarity Node-S units against the tried and trusted BAM. Along the way, we had the opportunity to perform a collocation analysis with several other low-cost sensor-based monitors. A traditional collocation analysis places one or more monitors in the same location as a regulatory grade monitor in order to assess and, often, correct for differences.
On May 24, 2019, the Shair team deployed 43 Clarity Nodes at a CARB rooftop monitoring location in Sacramento, CA and recorded the results (3 of these devices were later removed during analysis based on outlying data). In addition to our Clarity Nodes, this collocation included one BAM, two Environmental BAMs (eBAM) — a more portable BAM intended to produce reliable estimates on a daily average basis rather than an hourly or minute-by-minute basis— and several Purple Air low-cost-sensor-based monitors. Collocation was concluded on July 5, 2019.
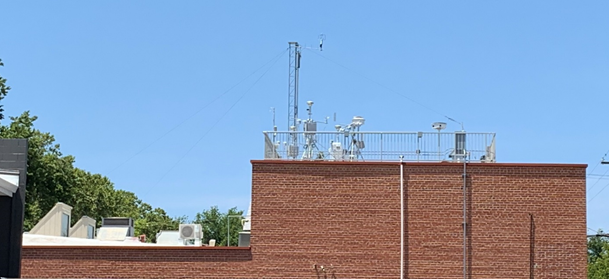
CARB’s T Street location rooftop monitoring location in Sacramento, CA – where the collocation was performed.
What was this collocation designed to achieve?
The original goal of this study was to collect air quality data using Clarity Nodes and determine how well they perform compared to the BAM. To our surprise, we were fortunate that the rooftop monitoring station happened to play host to an eBAM (Environmental Beta-Attenuation Monitors) and 6 PurpleAir monitors to which CARB allowed us data access. This allowed us to take part in a collocation analysis in which the readings of Clarity Nodes, BAMs, an eBAM, and several PurpleAir monitors could all be compared. eBAM monitors are marketed as a portable, scalable derivative of the BAM. PurpleAir is a low-cost sensor based air quality monitor that collects data using the same internal sensor hardware as the Clarity Nodes — for this reason, having a set of PurpleAir monitors allowed for a sanity check of our Clarity-BAM comparison data as well as an opportunity to see how our monitor compared to another widely popular low-cost sensor-based monitor. We did not expect much difference given that they use the same sensor. Conversely, the eBAM Monitors collect data using a beta-attenuation technique, which is expected to result in slightly different measurements of concentration than the Clarity Nodes and PurpleAir. In theory, this monitor operates similarly to the BAM, but is often observed as less precise, especially at lower pollution concentrations.
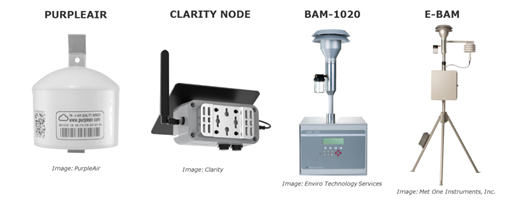
Comparing Each Low-Cost Sensor to the BAM
The Shair and Groundwork teams installed Clarity Nodes on the rooftop of CARB’s T Street location in Sacramento, CA and collected data from May 24th to July 5th, 2019. These Clarity Nodes took measurements approximately every 17 minutes. The 6 PurpleAir monitors to which we had data access collected data at a faster average sampling rate of once every 2 minutes, while the eBAM and the regulatory grade BAM provided readings representing an hourly average. (Note: For this analysis, we isolated the “primary” data stream from Channel A from each Purple Air air quality monitor)
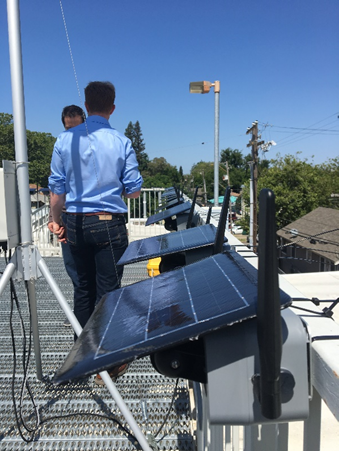
Clarity Node-S monitors lined up along CARB’s rooftop railing as part of the collocation.
As per the original intention of our collocation, data collected by the Clarity Nodes were made into hourly average values and then compared to hourly data produced by the BAM. As an added bonus of the collocation study, we were able to compare hourly averaged data from the PurpleAir monitors and data from the eBAM to hourly BAM output. For the purpose of this blog post, we report preliminary results (subject to change) from a single month-long period during this collocation: June 5, 2019 through July 5, 2019 (UTC). The figure below shows the concentrations measured by all relevant Clarity Nodes and PurpleAir monitors as well as the eBAM and BAM over this month-long period.
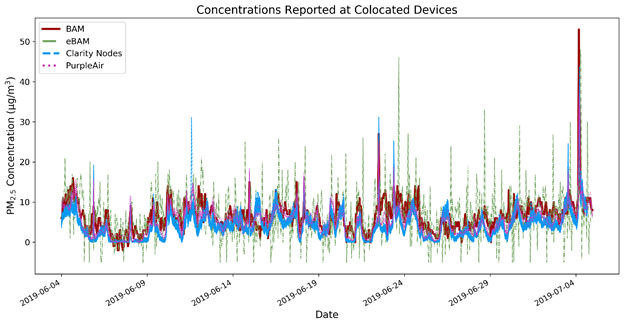
PM2.5 concentrations measured by the Clarity Nodes, PurpleAirs, eBAM, and BAM over a month during the collocation. Note the impressive spike in concentrations around July 4th (fireworks and grills!).
The Shair team determined minimum, mean, and maximum hourly average concentrations for the Clarity, PurpleAir, and eBAM monitors, normalized to the respective regulatory monitor with a simple multiplicative scaler. The PurpleAir monitor had the strongest correlation to the BAM, with an average r2 value of 0.65. Despite the use of the same internal sensor, the Clarity Node units demonstrated a considerably lower average r2 value of 0.52. The eBAM unit that we used in this analysis showed particularly poor hourly correlation with BAM output with an r2 of 0.09. (However, an analysis of daily correlation was, as expected, much higher at r2 = 0.88. Note: we would also expect the r2 values for the BAM comparisons of both the PurpleAir and the Clarity Node-S monitors to increase when compared at the daily average scale.)
As with any study, it is important to be aware of the major sources of error. Generally, air quality monitors are able to record measurements more accurately when ambient concentrations are relatively high (> 10 ug/m3, for example) In this case, the ambient concentrations of particulate matter in the Sacramento air were relatively low at about 6 ug/m3 on average, which is ultimately good for the residents of Sacramento, but proved to be a source of trouble for our study. It appears all monitors were affected by this issue, especially the eBAM, which is known to produce particularly low-precision hourly estimates.
An Unexpected Outcome
The discrepancy observed between the correlation of PurpleAir monitors to BAM data (r2 = 0.65) and the correlation of Clarity Nodes to BAM data (r2 = 0.52), which use the same sensor hardware to determine particulate matter concentrations, is unintuitive. In general, PurpleAir performed better against the BAM than the Clarity Nodes. Why?
With all the additional data we obtained from the rooftop in Sacramento, we decided to dig into the differences between the Clarity Nodes to the PurpleAir monitors. Addressing this discrepancy early in the process allowed us to apply our findings before the Richmond study was completely underway. One major difference that we observed between the two monitors is the sampling frequency. The number of samples a sensor collects every hour is a major contributor to the quality of the data recorded — more samples per hour will reduce error but also produce a more temporally-representative average of the concentration during that hour. The sensors in the PurpleAir sampled the air almost nine times more frequently than those in the Clarity Nodes.

The distribution of correlations (r2 values) between hourly average estimates produced from individual monitors as compared to BAM output and delineated by type.
As expected, we found that higher sampling frequencies resulted in less error during hourly averaged assessments. Given their higher sampling frequencies, PurpleAir devices may produce more reliable average hourly concentrations than Clarity Nodes.
To confirm our suspicion that the sampling frequency was affecting the results, we ran a simulation exploring what would happen if the PurpleAir device sampled about as frequently as the Clarity Nodes. To do this, we created a new artificial hourly average, which included only one out of every nine PurpleAir samples. This set the PurpleAirs’ effective sample rates at about the same value as the Clarity Nodes’. We then ran the same correlation analysis and found that the correlation between the new “resampled” PurpleAir and the BAM was lower than it was previously. In fact, the average r2 of the PurpleAir correlation to the BAM decreased to 0.53 — nearly identical to the average r2 of the Clarity Node. Additionally, as shown in the below figure, the standard deviation of r2 across PurpleAir devices increased to more closely match that of the Clarity Nodes.

The distribution of correlations (r2 values) between hourly average estimates produced from individual monitors as compared to BAM output and delineated by type with the addition of a new “type” representative of PurpleAir monitors with their original data resampled to more closely match the sampling frequency of the Clarity Nodes.
We then took this one step further and simulated data from PurpleAir data by skipping between 1 (i.e., taking every second measurement) and 29 measurements per “sample.” The goal of this assessment was to explore whether a larger trend exists between sample frequency and correlation of hourly averages with BAM output. This result is shown in the figure below. As the sample frequency decreased (toward the right), the correlation with BAM output decreased (p < 0.01 when linearly tested. It should be noted that a non-normality of the data is indicated by a Shapiro-Wilk test [p < 0.001]; more analysis is needed to confirm the significance of this inverse trend).

The average correlation between PurpleAir hourly average concentrations and BAM output as sample frequency is reduced (i.e. as the number of skipped data points per sample increases). The first point (from the left) is the original PurpleAir average r2 . The relationship is inverse (p < 0.01, r2 =0.24, RMSE= 0.03).
Conclusion
Our co-location at CARB’s T Street monitoring location was a great success.
Monitor reliability
First and foremost, our collocation analysis demonstrated a reasonable correlation between the Clarity Node-S monitors that we are using in Richmond and the tried, trusted, and far-more expensive regulatory grade Beta Attenuation Monitor (BAM; r2 = 0.52). Correlations spanned a range that included the level of correlation observed between two BAM units collocated at a regulatory monitoring site in Vallejo several years ago (r2 = 0.58),(1) and suggest reasonable device sensitivity at ambient pollution concentrations and the potential for calibration. While not explicitly discussed in this write-up, Clarity devices also showed high correlation with each other, suggesting low inter-device variability, indicating that individual Clarity monitors should respond similarly to varying levels of PM2.5. This has implications for various collocation strategies, especially one in which a realtime collocation-based correction factor is developed for a single Clarity monitor and applied to other Clarity monitors in the region.
Same sensor, different results
We also uncovered a major discrepancy between the “reliability” of the Clarity Node and the PurpleAir (as assessed via correlation between hourly averaged monitor data and BAM output) despite their use of the same internal sensing hardware. Clarity Node correlations with the BAM were, on average, 0.52 while PurpleAir correlations with the BAM were about 25% higher with an average correlation of 0.65. After some experimentation with the data, we discovered the discrepancy to be related in large part to the number of samples taken every hour — more samples in an hour typically resulted in a higher reliability. When the PurpleAir data were made to mimic the Clarity Node’s sampling frequency, their correlation with BAM data became much more similar to the Clarity Node, dropping to an average correlation of 0.54.
Findings that improve the Richmond study and inform future study design
The results of this down-sampling simulation suggest that the Clarity Node units may substantially increase their reliability (r2 vs. the BAM) by increasing their sample frequency and, as a result, may be made to better represent true pollution conditions. This finding proved to be a major milestone in the study. Consequently, we’ve been working with Clarity to increase the sampling frequency of the monitors located around Richmond to improve the quality of their results.
Moving beyond critical limitations
PM2.5 concentrations were particularly low during the collocation measurement period, averaging approximately 6 ug/m3 — a value just barely above the BAM hourly detection limit of approximately 4.8 ug/m3 (2) and likely at or below the reliable detection limits of the low-cost sensors used in the Clarity Node and Purple Air. We expect to see ambient PM2.5 concentrations that exceed these values, and so future collocation testing of the Clarity Node should be done under higher concentrations to better understand the reliability of these devices under conditions that maybe more typical in the Richmond area. One idea moving forward might be to establish long-term collocations of a handful of Clarity Nodes throughout the study area.
A big win for AB617
This project sets precedence for future AB617 sensor-based projects by demonstrating the utility that can come from a pre-study collocation trial. From quantifying the general reliability of two popular low-cost sensor-based monitors to shedding light on sample frequency best practices, this work specifically adds great value to the community-oriented work currently underway in Richmond. We are excited to apply what we’ve learned here to improve this and future AB617 work.
Acknowledgements and References:
Special thanks to the CARB Advanced Monitoring Techniques Section (AMTS) Monitoring and Laboratory Division for allowing us to utilize their space and data, to David Lu of the Clarity Team for providing support during the deployment visit, and to Justin Bandoro of Ramboll Shair for his assistance with quality assurance in the analysis. The authors are employees of Ramboll, which is subcontracted for technical expertise on the Groundwork Richmond Air Rangers grant.
1. Holstius et al. 2014. Field calibrations of a low-cost aerosol sensor at a regulatory monitoring site in California. Atmospheric Measurement Technology. 7:1121–1131. doi:10.5194/amt-7–1121–2014. Available at < https://www.atmos-meas-tech.net/7/1121/2014/>.
2. https://metone.com/wp-content/uploads/2019/04/bam-1020_detection_limit.pdf
Update: an earlier version of this blog stated “ These devices are very expensive — upwards of $500,000 for a BAM when accounting for maintenance, according to a recent presentation given by a member of the Bay Area Air Quality Management District. It is not economically feasible to implement a BAM on every street corner. Low-cost sensors that perform well against regulatory grade equipment open the door for everyone, including those in disadvantaged communities, to access real-time, local air quality information.” This has been updated for clarity and to more appropriately fit the article’s context.